PUBLICATION EXCLUSIVE: Achieving refractive accuracy in cataract surgery
A large meta-analysis of many years’ worth of LASIK studies showed that 99.5% of patients achieved an uncorrected vision of 20/40 or better and 91% of patients achieved 20/20 or better. These 91% of patients were corrected to within ±0.5 D of plano or the intended target. In comparison, cataract surgery is not nearly as good, with only about 60% of patients achieving the same result. Published guidelines for cataract surgeons list 55% as the appropriate target. Surely, we can do better than this.
There have been a lot of improvements in the expensive machines that we use to measure the eye. Going from ultrasound A-scan to optical coherence biometry improved the accuracy of axial length measurement dramatically, by up to 10 times, but that did very little to improve the refractive outcomes in most cases. We are now in the age of microscope-mounted intraoperative aberrometers, and I am in the midst of trying the newest generation of these devices. I must admit, however, that I am disappointed in the initial results, and it strikes me as odd that these devices all request so much input data. If these devices are so accurate, then why do I have to enter any data? Does your autorefractor require you to enter the current prescription before measuring the new one?
The answer is in the mathematics. Our biometry, whether done preop or intraop, is accurate, but the way that we use this data is not as good. In the past we used decision trees to help decide which of the many IOL formulae we should use in a particular case. That was cumbersome and has since transformed into three main approaches: using the sweet spots of multiple formulae (Barrett Universal from Graham Barrett, MD); using an artificial intelligence approach (Hill RBF from Warren Hill, MD); and using a novel way to graph the sweet spots of all formulae, hone the results with big data and incorporate artificial intelligence to constantly improve (Ladas Super Formula from John Ladas, MD, PhD).
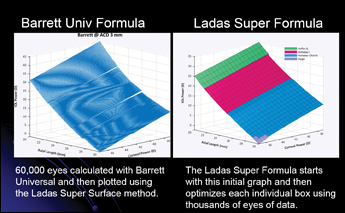
Images: Devgan U
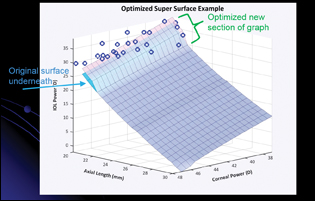
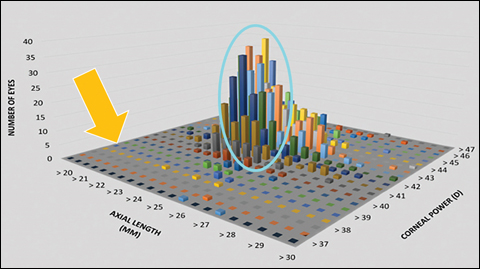
Barrett Universal and Hill RBF
Approach one is the Barrett Universal formula, which seems to divide the continuum based on axial lengths as determined by an analysis of 60,000 eyes of data points using this formula and then plotted using the Ladas Super Surface 3-D graphing technique. The details and specifics of the Barrett formula have not been publicly shared to my knowledge. The Barrett Universal is a good choice and certainly better than relying on a single older formula. A published study showed that as is, the Barrett formula can give accuracy of about 80% within ±0.5 D of target.
Approach two is the Hill Radial Basis Function network, which is a type of mathematical modeling that uses a neural network to approximate IOL power based on input parameters, pattern recognition and machine learning. It states that it is specifically intended for a single biometer and a single IOL design and that it may not work the same way with other IOLs or biometers. Artificial intelligence neural networks are used in many other aspects of business, such as handwriting recognition at banks and post offices, where they can function with greater than 99% accuracy. The idea behind “big data” is that machine learning can take place as more and more data are entered. My own personal experience with the Hill RBF is that it works well with the specific IOL for which it is designed.
- Click here to read the full publication exclusive, Back to Basics, published in Ocular Surgery News U.S. Edition, February 25, 2017.