Breaking down the sham of statistical significance
The ophthalmology community should not rely on P values, according to Jack S. Parker, MD.
Thomas Bayes died in 1761, but his theorem lives on and is inspiring a new generation of statistical thinking. Whether you are frequentist or Bayesian, Jack S. Parker, MD, shares a compelling argument for us to ditch the P < .05 philosophy.
Anthony Khawaja, PhD, FRCOphth Chair of the SOE Young Ophthalmologists committee
What does P < .05 actually mean? I am asking you, the reader, right now. This is not a rhetorical question. Take just a moment, and really try to formulate an answer.
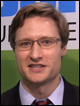
If you said: “P < .05 means that there is less than a 5% chance that a study’s results are due to randomness alone,” then you are wrong. And not just wrong in a semantic, pedantic or quibbling sort of way. You are spectacularly, blindingly and egregiously wrong; wrong in a way that reflects a complete misunderstanding of the statistical concept.
You are also wrong if you said: “There is a 95% chance that the study’s findings are true/the null hypothesis is false/the observed results will be replicated.” In fact, according to how the average experiment is powered, less than half of all studies with P < .05 will have their results replicated if run a second time.
All of this should concern you, that — despite years of accumulated encounters with this mathematical index and its ubiquitous presence in nearly every major ophthalmology journal — it is totally unclear, to basically everyone, what this metric actually means.
Somehow, the story gets even worse. Not only are the concepts of P values and statistical significance confusing and obscure, but — even properly applied and understood — they contain almost no practically useful information. Specifically, they do not distinguish between “real” and “random” results, are conspicuously absent from the journals of the “hardcore” sciences such as physics and chemistry, and have been the ongoing public target of professional statisticians for decades. So what is going on? What is a P value? And if it is so bad, why is it everywhere in medicine?
What is a P value?
The first thing to know is that the starting presumption of all tests for statistical significance is that the null hypothesis is true: namely, that a proposed intervention has no effect. P values answer this question: Assuming that the null hypothesis is true, how often would results as extreme as the study’s appear? What P values provide is the probability of the data given the hypothesis of pure randomness, not the probability of the hypothesis (that the intervention has no effect) given the data. In other words, P values tell you the opposite of what you want to know. P < .05 simply indicates that the observed results are rare, not that they were not randomly generated. Therefore, isolated P values are unable to distinguish the effects of an intervention from random chance. This failure alone is enough to recommend abolishing their use, especially considering that this tool — while mostly unhelpful — is nevertheless frequently mistaken as the single most important indicator of a study’s scientific validity. But beyond even this, other problems with the practice of testing for statistical significance abound, including:
1. It makes for weak and pitiful hypotheses. Theorizing that a particular intervention may have “some” effect on another thing is a sad and feeble proposition because “some” might mean “so little it is irrelevant” and because these sort of hypotheses are too easy to prove because every action, no matter how trivial, probably has some imperceptible effect on everything else. In other words, P values detract from the real statistical heroes: effect sizes. And arguably, if there is to be some statistical requirement for publishing in the top journals, it should be that a studied intervention has at least some amount of effect (ie, potential to be important) rather than at least some P value.
2. It does not add anything. Good science demands that experiments be replicated. Results due to randomness are therefore likely to be revealed as such, anyway, without the need to pretend we can distinguish “real” vs. “random” using a single statistical tool.
3. Better alternatives exist. These include confidence intervals and Bayesian regression analyses, both of which are proudly embraced by the global community of professional statisticians, rather than P values, which are publicly flogged in their academic circles year after year.
But if all this is true, then why are tests of “statistical significance” so popular? The short answer is that P values are convenient. They are simple to calculate, and they vaguely relate to the relevant question: How much randomness do a study’s results reflect? They also offer what appears to be a simple dichotomous yes/no test for determining which results can be believed. But, unfortunately, our community’s bizarre obsession with P values has done far more harm than good: by instilling a false confidence (or skepticism) in the truth of our results, by distracting us from effect size, by downplaying the importance of replication, and — perhaps worst of all — by convincing our colleagues in physics and chemistry that we do not understand basic math.
- Reference:
- Ziliak ST, McCloskey DN. The Cult of Statistical Significance: How the Standard Error Cost Us Jobs, Justice, and Lives. Ann Arbor, Mich.: University of Michigan Press; 2008.
- For more information:
- Jack S. Parker, MD, a YO member of the OSN Europe Edition Board, can be reached at Parker Cornea, 700 18th St. S #503, Birmingham, AL 35233, USA; email: jack.parker@gmail.com.
Disclosure: Parker reports no relevant financial disclosures.