Machine learning identifies three CKD subgroups for more precise risk stratification
Click Here to Manage Email Alerts
A machine learning algorithm grouped patients with chronic kidney disease into three distinct subgroups. Each subgroup was associated with different risks for disease progression, cardiovascular events and mortality.
“There are clear differences across individuals with CKD that can be captured by a comprehensive examination of phenotypic data, such as laboratory results, medical history, medications and social factors,” Zihe Zheng, MBBS, MHS, of the department of biostatistics, epidemiology and informatics at Perelman School of Medicine at the University of Pennsylvania, and colleagues wrote. “More sophisticated phenotyping may reveal different and more reliable CKD clusters and underlying disease pathologies, which could help better understand different mechanisms for disease pathways and progression.”
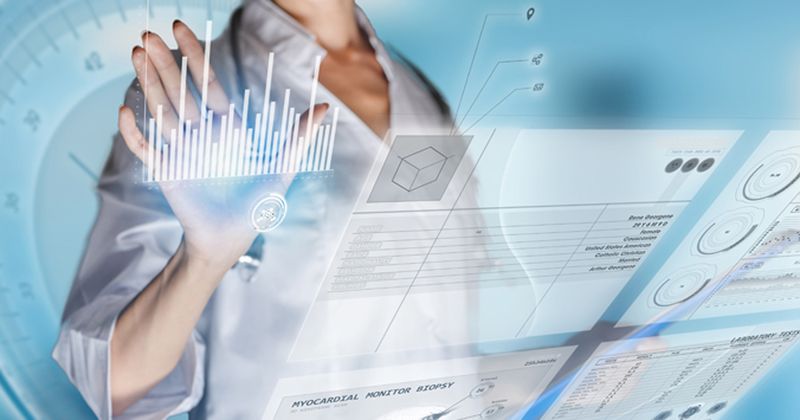
To this end, including 2,696 participants from the Chronic Renal Insufficiency Cohort study (CKD stages 2 to 3), researchers applied “machine learning consensus clustering to multidimensional patient data.” Considered data included demographics, biomarkers from blood and urine, health status and behaviors, and medication use (72 baseline characteristics in total).
The algorithm categorized patients into three distinct subgroups.
According to Zheng and colleagues, patients assigned to cluster one had “relatively favorable levels of bone density and cardiac and kidney function markers,” were less likely to have diabetes or obesity, and used fewer medications. Those in cluster two had a higher prevalence of diabetes and obesity and used more medications, while those in cluster three had “less favorable levels of bone mineral density, poor cardiac and kidney function markers, and inflammation.”
After controlling for established CKD risk factors (including eGFR, urine albumin-to-creatinine ratio, blood pressure and diabetes), researchers found each cluster was significantly associated with the risks of CKD progression, kidney failure requiring replacement therapy, cardiovascular disease outcomes and mortality (cluster one, low risk; cluster two, medium risk; cluster three, high risk).
“Phenotyping of patients with CKD using unsupervised machine learning algorithms provides a powerful tool for identifying patient heterogeneity,” Zheng and colleagues concluded. “Future studies are required for external validation of the identified clusters and to investigate CKD subgroups by incorporating novel biomarker data (eg, genomics, metabolomics, proteomics, etc.) as an important next step toward identifying subgroup-specific therapeutic targets for better patient care and precision medicine in nephrology.”