Machine learning with cardiac biomarkers may help predict atrial fibrillation in CKD
An approach that uses artificial intelligence by including cardiac biomarkers into a machine learning algorithm more accurately predicted atrial fibrillation in patients with chronic kidney disease than use of a previously published model.
“Clinically available biomarkers of myocardial injury (high-sensitivity troponin T [hsTnT]) and hemodynamic stress (N-terminal brain natriuretic peptide [NT-proBNP]) are strongly associated with atrial fibrillation in chronic kidney disease and have been included in AF prediction models in community-based populations,” Leila Zelnick, PhD, of the University of Washington, and colleagues wrote a poster presented at ASN Kidney Week. “We investigated the incremental prognostic value of NT-proBNP and hsTnT for AF prediction compared to standard clinical variables in CKD patients enrolled in the Chronic Renal Insufficiency Cohort (CRIC) using machine learning methods.”
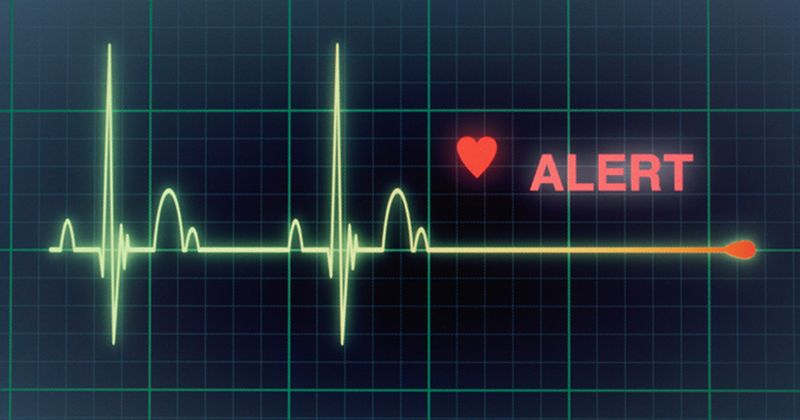
For the study, researchers included 2,690 participants who had no prior history of AF (mean eGFR, 45 mL/min/1.73m2). They compared the discriminatory ability in predicting incident AF between the machine learning model and a previously validated clinical prediction model (CHARGE-AF).
During 7.3 years of follow-up, 251 incident AF events occurred, with researchers finding the CHARGE-AF prediction equations had a cross-validated C-index of 0.69. The model based on machine learning improved the C-index to 0.75, 0.73 and 0.76, respectively, when adding NT-proBNP, hsTnT or both biomarkers.
In a related press release, Zelnick commented on how this pairing of cardiac biomarkers and machine learning might be used in real-world settings.
“The application of such a model could be used to identify patients with CKD who may benefit from enhanced cardiovascular care and also to identify selection of patients for clinical trials of AF therapies,” she said.