EHR-integrated model may accurately predict AKI risk in hospitalized patients
Researchers successfully integrated a risk algorithm into the electronic health record at an urban, tertiary-care hospital. The algorithm accurately predicted which patients would go on to develop AKI within 24 hours.
“AKI is most often recognized by an increase in serum creatinine, a late marker of the syndrome,” Ugochukwu Ugwuowo, MBBS, of Yale University School of Medicine, and colleagues, wrote. “As no single therapy has been shown to improve AKI outcomes once the creatinine has started to rise, there has been substantial interest in identifying earlier biomarkers for AKI.”
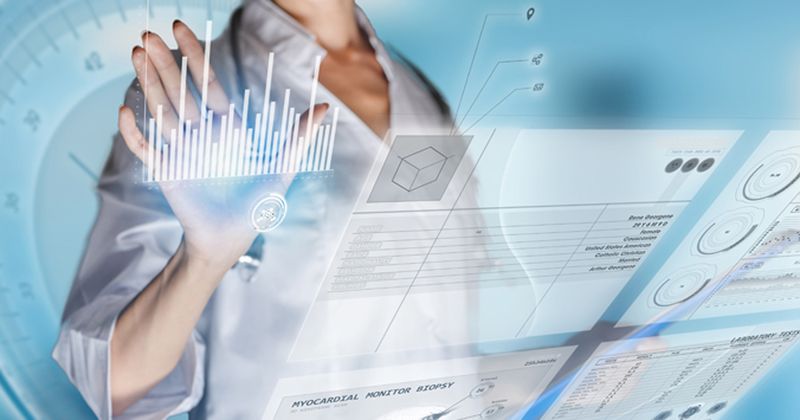
Despite the utility of biomarkers, the researchers contended that high costs accompany biomarker measurement and there is difficulty in clinical interpretation of the results, thus limiting the adoption of biomarkers as a common prediction strategy.
“Given the recent advancements in the electronic health record (EHR), data accessibility and prognostic modelling, ‘electronic’ biomarkers – statistical models driven by real-time EHR data – may provide a mechanism to target diagnostic and therapeutic interventions to a general hospitalized population,” they suggested. “However, all of these studies are based on retrospective data and do not evaluate the multitude of challenges faced when implementing a model in the EHR and prospectively predicting AKI in real-time.”
To gain experience with “real-time” AKI prediction, the researchers conducted a prospective observational cohort study of 2,856 hospitalized adults, using an EHR-integrated model which continuously calculated AKI risk.
According to the researchers, the risk was updated throughout the hospital stay as new information was entered into the EHR, with the algorithm generating a “pre-AKI” alert at the first instance of a patient crossing the 15% risk threshold (alerts were delivered to the study team, but not to treatment teams).
AKI within 24 hours of the pre-AKI alert was considered.
At the time of the alert, the mean predicted probability of AKI was 19.1%. Researchers found 18.9% of patients went on to develop the condition within 24 hours of the alert (median time to AKI, 10 hours).
“We demonstrated that the AKI alert could be used in real-time to target biomarker measurements to patients,” they wrote. “Our results, though specific to the convenience sample of patients enrolled, generate intriguing hypotheses.”
The researchers argued that examination of the findings suggests that AKI “may not follow a discrete renal insult (as seen in studies of patients after heart surgery for example) but occur on a spectrum that begins with pre-renal stress and subsequently progresses to severe AKI and its downstream consequences.”
Therefore, they recommend looking into interventions that restore renal perfusion.
“Future studies are warranted to determine whether prognostic models may be used to increase the window of opportunity to prevent AKI development and improve patient outcomes,” they added.