AI machine-learning tool may help predict risk for burn-related AKI
Click Here to Manage Email Alerts
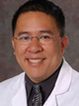
A tool that uses artificial intelligence and a machine learning algorithm enhanced the performance of existing tests for predicting acute kidney injury in certain high-risk populations, according to a published study.
“Up to 58% of burn patients acquire AKI due to pre-renal (eg, burn shock, sepsis) and renal mechanisms (eg, nephrotoxic medications) of injury — with AKI common within the first week due to inadequate resuscitation during the critical first 24 hours of admission,” researchers from the University of California, Davis wrote. “Despite this high prevalence, early recognition remains challenging due to the reliance on serum/plasma creatinine and urine output for diagnosing and staging AKI — biomarkers that have known limitations.”
“AI and machine learning are the future,” Nam K. Tran, PhD, FACB, associate professor of clinical pathology and director of clinical chemistry and point-of-care testing at the university, told Healio/Nephrology. “It may not replace humans, but it is already a huge part of our daily lives.”
Employing the Scikit-Learn’s k-nearest neighbor algorithm, researchers developed machine learning models. The performance of the models for improving AKI recognition was evaluated using an existing database of 50 adults who were hospitalized for having at least 20% total body surface area burns. During the first 24 hours of hospital admission, neutrophil gelatinase associated lipocalin (NGAL), urine output, creatinine and N-terminal B-type natriuretic peptide (NT-proBNP) were measured.
Researchers found 50% of burn patients experienced AKI within the first week following admission with the machine learning models that contained NGAL, creatinine, urine output and NT-proBNP achieving 90% to 100% accuracy for identifying AKI. For the machine learning models that contained only NT-proBNP and creatinine, 80% to 90% accuracy was observed.
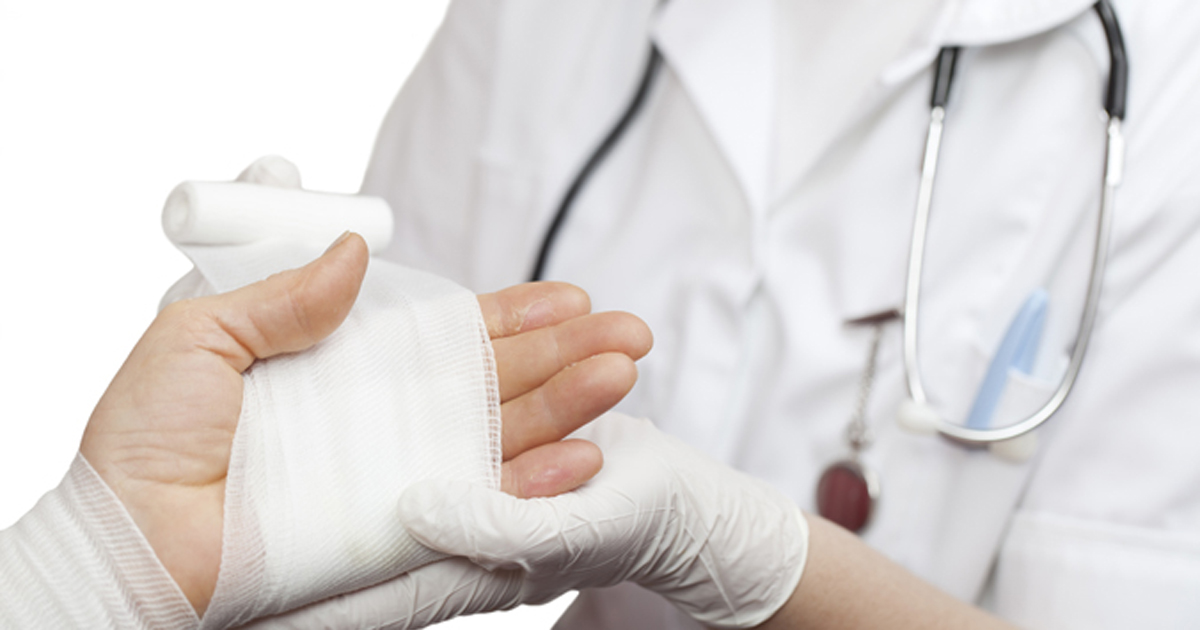
Researchers further determined that, while mean time to AKI recognition was 42.7 hours after hospital admission when using urine output and/or creatinine alone, the machine learning algorithm recognized AKI within 18.8 hours.
Tran also told Healio/Nephrology that, expanding on a previous study conducted by UC Davis researchers that found plasma NGAL testing to be effective for AKI detection, the machine learning models recognized AKI at least a day earlier with a single measurement.
“Presently, NGAL is not FDA approved,” Tran said. “So, this AI approach can improve performance of plasma creatinine and urine output as AKI biomarkers.”
Tran concluded, “We have to also be aware that an AI that works at one institution may not work well at another. Tests may differ significantly between hospitals, as well as populations. AI has a lot to promise, but we also need to learn more and implement it in a rational way.” – by Melissa J. Webb
Disclosures: The authors report no relevant financial disclosures.