Machine-learning algorithm predicts severe sepsis in hospital patients
An algorithm using artificial intelligence can foresee severe sepsis and septic shock in hospital patients, giving clinicians a potentially lifesaving edge, according to researchers.
The so-called “machine-learning algorithm” identifies patients at risk by processing their electronic health records, they reported at the American Thoracic Society International Conference in Washington, D.C.
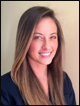
“We have developed and validated the first machine-learning algorithm to predict severe sepsis and septic shock in a large academic multi-hospital health care system,” study researcher Heather Giannini, MD, an internal medicine resident at the Hospital of the University of Pennsylvania in Philadelphia, said in a news release. “This is a breakthrough in the use of machine-learning technology and could change the paradigm in early intervention in sepsis.”
Machine learning allows computers to detect patterns in data without being programmed to do so. Researchers have previously consulted EHR data to detect the start of sepsis or general declines in patients’ conditions.
To test their own algorithm, Giannini and colleagues trained a random forest classifier — a method of classifying a wide range of information — to use EHR data, including lab samples, vital signs and demographics. They applied the algorithm to data from 162,212 patients who had been discharged from three University of Pennsylvania acute-care hospitals between July 2011 and June 2014.
The algorithm identified patients as having severe sepsis or septic shock 12 hours before onset. Altogether, 943 (0.58%) patients met the criteria for either condition.
The researchers said they then validated the algorithm in real time among 10,448 patients. In all, the algorithm produced 314 alerts to either condition. About 3% of all acute-care patients screened positive for severe sepsis or septic shock, and 10 alerts were activated per day among the three hospitals.
Sensitivity and specificity for detecting patients with severe sepsis or sepsis shock were 26% and 98%, respectively, the researchers said.
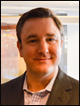
“We were hoping to identify severe sepsis or septic shock when it was early enough to intervene and before any deterioration started,” study researcher Craig Umscheid, MD, MSCE, an associate professor of medicine at the Hospital of the University of Pennsylvania, said in the news release. “The algorithm was able to do this. This is a breakthrough in showing that machine learning can accurately identify those at risk of severe sepsis and septic shock.”
Umscheid said researchers must next determine whether the algorithm is clinically effective. – by Joe Green
Reference:
Giannini HM, et al. Abstract 7248. Presented at: The American Thoracic Society International Conference; May 19-24, 2017; Washington, D.C.
Disclosures: Infectious Disease News was unable to confirm the researchers’ relevant financial disclosures at the time of publication.