New model predicts HCV progression, liver-related events
A risk prediction model developed by researchers from University of Michigan Health System, helped identify which patients with hepatitis C virus infection have the most urgent need for new anti-viral treatment, according to newly published data in Hepatology.
“Offering immediate treatment to patients identified as high risk for poor health outcomes would allow these patients to benefit from highly effective treatments as other patients continue to be monitored and their risk assessment updated at each clinic visit,” Monica Konerman, MD, MSc, gastroenterology fellow at the University of Michigan Health System, said in a press release.
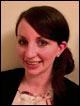
Monica Konerman
The researchers, in collaboration with colleagues from VA Ann Arbor Health Services Research and Development Center of Clinical Management Research, analyzed clinical data of HCV patients, such as age, body mass index and routine lab measurements, from the HALT-C clinical trial, to estimate patients’ risk of progression of liver disease. Logistic regression and the random forest and boosting machine learning methods were used to construct the models and predict outcomes over 12 months, according to the research.
Results showed that the longitudinal models performed better than baseline models for both fibrosis progression and liver-related clinical outcomes, such as mortality, liver transplant and hepatocellular carcinoma (P < .0001). In addition, the longitudinal machine models had negative predictive values of 94% for both of these outcomes.
The area under the receiver operating characteristic curve (AUROC) for longitudinal models of fibrosis progression was 0.78 (95% CI, 0.74-0.83) using logistic regression, 0.79 (95% CI, 0.77-0.81) using random forest and 0.79 (95% CI, 0.77-0.82) using the boosting method.
AUROC for longitudinal models of clinical progression was 0.79 (95% CI, 0.77 - 0.82) using logistic regression, 0.86 (95% CI, 0.85-0.87) using random forest and 0.84 (95% CI, 0.82-0.86) using the boosting method.
The risk prediction model could potentially be added to an existing electronic medical record as a health care decision guide for doctors, according to the release, and can also establish how often patients attend doctor visits or undergo monitoring tests.
“Ideally, we would treat all patients,” Konerman said. “Until logistic and financial barriers are solved, clinicians and policy makers are faced with trying to target these therapies to patients with the most urgent need. The model allows us to identify these patients with greater accuracy.” – by Melinda Stevens
Disclosure: Konerman reports no relevant financial disclosures. Please see the full study for a list of all other authors’ relevant financial disclosures.